What Is a Logical Data Model?
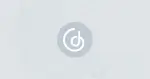
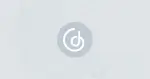
A logical data model (LDM) is a type of data model that describes data elements in detail and is used to develop visual understandings of data entities, attributes, keys, and relationships. This kind of model is uniquely independent from a specific database in order to establish a foundational structure for components of the semantic layer in data management systems. Think of an LDM as a blueprint: It represents the definitions and characteristics of data elements that stay the same throughout technological changes.
Definition of Data Modeling
Before we dive deeper into LDMs, what is a data model — and data modeling — anyway? According to IBM, it is "the process of creating a visual representation of either a whole information system or parts of it to communicate connections between data points and structures." Being able to visualize these relationships between data structures gives organizations the ability to determine which areas of business need improvement.
There are three types of data models: conceptual, logical, and physical.
1. Conceptual data models: defines what the system contains
Typically, data architects and business stakeholders are the users who create conceptual data models. These types are built with the intention to organize and define business concepts and rules. They have different types of submodels, such as the semantic data model and the business data model.
2. Logical data models: defines how a system has to be implemented and is not specific to a database
Users who generally create LDMs are data architects and business analysts. These models are used as a foundation for physical data models because they distinguish the relationships and attributes for each entity.
3. Physical data models: how the system will be implemented to a specific database management system
This is where the database designers and developers come in to create physical data models that are expansions of the logical and conceptual models. These models are designed for business purposes. You can read more in further detail here.
Why not try our 30-day free trial?
Fully managed, API-first analytics platform. Get instant access — no installation or credit card required.
Get startedLogical Data Model Examples
Below are a few examples of LDMs found from a variety of sources. You’ll probably notice pretty quickly that while the design of the LDMs can look different, the template and arrangement of figures are the same. You can see different components of the data model, too, including datasets (boxes) and the connection between them (arrows).
This is an LDM example created by the GoodData LDM Modeler:
In the GoodData LDM Modeler, you can distinguish what type of component you are handling by the color label. The green datasets represent facts; the yellow datasets are the attributes; and the blue dataset represents the date.
Here is an LDM example from 1keydata:
This simply designed LDM includes informational elements of normal, day-to-day business. You can access the time, product, and store datasets.
Here is Lucidchart’s LDM example:
This might be what the logical data model looks like for a government facility. It includes state, city, and county datasets that can be looked up per employee. From there, you can follow the arrows to see the connection from employee data to the employer cross-reference datasets.
Tibco has this example of an LDM:
Similar to the example by 1keydata, this model also illustrates the connections between elements of day-to-day business. The modern design template makes this model more appealing to the eye and easier to read.
Data Architecture in the GoodData LDM Modeler
Data architecture, according to CIO, refers to the storage, management, and maintenance of data in an organization. The goal of data architecture is to put business needs into system requirements and thus manage the data flow throughout an organization. For the GoodData platform, the LDM is used as a foundation for the semantic layer, which portrays the data structures and their relationships in a business-friendly way. With our SaaS model, all end users are able to understand the data in the same way, and our use of MAQL (multidimensional analytics query language) allows for composable data and analytics. As such, objects within the GoodData LDM Modeler can be reused numerous times to create data visualizations.
With the GoodData LDM Modeler, there are multiple components and modeling tools provided to help users display their data and easily modify them. The platform has two main functions: data loading and data querying. Data loading is the process of transporting data from a source or file and into a database. Data querying, on the other hand, is the request for data from the database to retrieve it. These functions help users in ensuring that the data is accurately filed into the correct dataset and then returned to the querying client.
In the GoodData platform, users have the option of creating multiple workspaces, or units where they can view dashboards and analyze data for different use cases or customers. The data modeling workspace is entirely integrated with the ability to bring together multiple datasets, customize them, and build relationships between the datasets. The LDM Modeler is also able to provide metrics and reporting capabilities from these defined connections between datasets.
The logical data model components in GoodData include:
Dates: can include day of the week, month, quarter, year, and more
Facts: numerical values or pieces of data
In the LDM Modeler, there can be three types of facts:
- Additive: facts used for calculations, such as adding together
- Non-additive: facts that cannot be summed together
- Semi-additive: facts that can be added, but only in certain contexts
Attributes: names of a characteristic or type of entity
A set of attributes is called a dimension, which is stored in tables. For example, “address,” “city,” “state,” and “zip code” may all be attributes that belong to a dimension labeled “location.”
Datasets: organizational units for set of facts and/or attributes
Relationships can be drawn between datasets to showcase associations. As mentioned previously, in the GoodData LDM Modeler, datasets are distinguished in boxes and by types with the color of the label.
Connection points: the primary keys for your database
Connection points are used for emphasizing uniqueness in a dataset. This process of building relations and connections between datasets is important in telling the system what can be sliced, and by what, when producing your own metrics.
Main Takeaways and How to Learn More
Data models are utilized by organizations to communicate relationships and connections between data in a visual manner. With these three types of data modeling — conceptual, logical, and physical — businesses are better equipped to identify areas of improvement (such as how to build semantic layers for massive scale analytics) based on more context of business situations.
In particular, logical data models give more details into data and its entities, providing visual context into data, attributes, and relationships within them. They serve as a blueprint for describing how a system has to be implemented, without being built into a specific database or database management system.
Creating data models for the first time can be a complex process with all the different components and principles of data architecture, and with common mistakes that can occur. At GoodData, we provide free online courses on understanding the logical data model that teach users in more detail all the elements in creating these figures, including a guide on how to navigate it in our platform.
Why not try our 30-day free trial?
Fully managed, API-first analytics platform. Get instant access — no installation or credit card required.
Get startedHeader photo by John Schnobrich on Unsplash