With the growing number of data analytics solutions available in the market, choosing the right option for your business can feel like an overwhelming task. Having a clear understanding of your unique use case and familiarizing yourself with terms commonly used in the data and analytics industry can narrow your options and simplify the decisionmaking process.
This guide will explain the terms that you need to understand in order to smoothly navigate the data and analytics industry. Additionally, it will explore the most important differences in use cases and how some major solutions in this field differ from one another. We hope this guide will make it easier to find what you are looking for.
Part 1: Important Terms to Know
Business Intelligence vs. Data Analytics
In today’s data-driven world, the terms business intelligence (BI) and data analytics have become nearly synonymous. Although strong overlaps between BI and data analytics exist, nuanced differences also set them apart.
Difference #1: The End User
While BI is specifically targeted at business end users with minimum or zero technical knowledge, data analytics can be more technical and targeted at a broader audience, including data scientists. The term data analytics often describes a solution for more technical end users who know how to query data themselves to search for insights in enormous, raw datasets.
Difference #2: The Purpose
BI primarily focuses on internal team consumption. In other words, internal business users — with the goals of optimizing processes and improving decision-making — observe data that is generated by daily operations. Though the distinction suggests that BI does not directly generate revenue for the business, it can still significantly enhance overall output.
In contrast, data analytics is often intended for external users. Data analytics can be added to an already existing software product (or service) to encourage adoption and to increase the product’s value. As a result, data analytics also can directly generate revenue for the organization who had purchased the analytics solution and implemented it within their product.
Typically, software as a service (SaaS) companies use embedded analytics as such and these use cases are sometimes referred to as customer-facing analytics. Overall, the differences between BI and data analytics are often subtle. There is no industry-wide consensus on the difference between the two, so as the industry continues to evolve, organizations may perceive or define them differently.
Despite the subtle differences between BI and data analytics, their goal remains the same: to turn data into visualizations that carry valuable information.
What Is Embedded Analytics?
For the most part, there is no difference between embedded analytics and other types of analytics.
Like other kinds of analytics, it transforms data stored at different locations into comprehensive charts and dashboards. The process flows from data integration to data transformation, data modeling, and data visualization.
What sets embedded analytics apart is that, as an individual software solution, it does not look and act as a standalone solution. Instead, it is integrated within another application, software product, or web portal.
That integration could simply serve aesthetic purposes on the surface or, alternatively, it could be incorporated into the foundation of a tool. There are different types of embedded analytics and they differ in three critical areas:
- Usability: What will your business end users be able to do with embedded dashboards and visualizations? Despite being embedded, will the dashboard be still dynamic and interactive? Will end users be able to drill into dashboards, remove a metric, filter, or change the type of visualization?
- Ease of Embedding and Customization: Will developers need to know the basics of coding and SDKs? How fast and developer-friendly will the implementation of embedding and the customization of dashboards be?
- Security: Is it safe to integrate with another application?
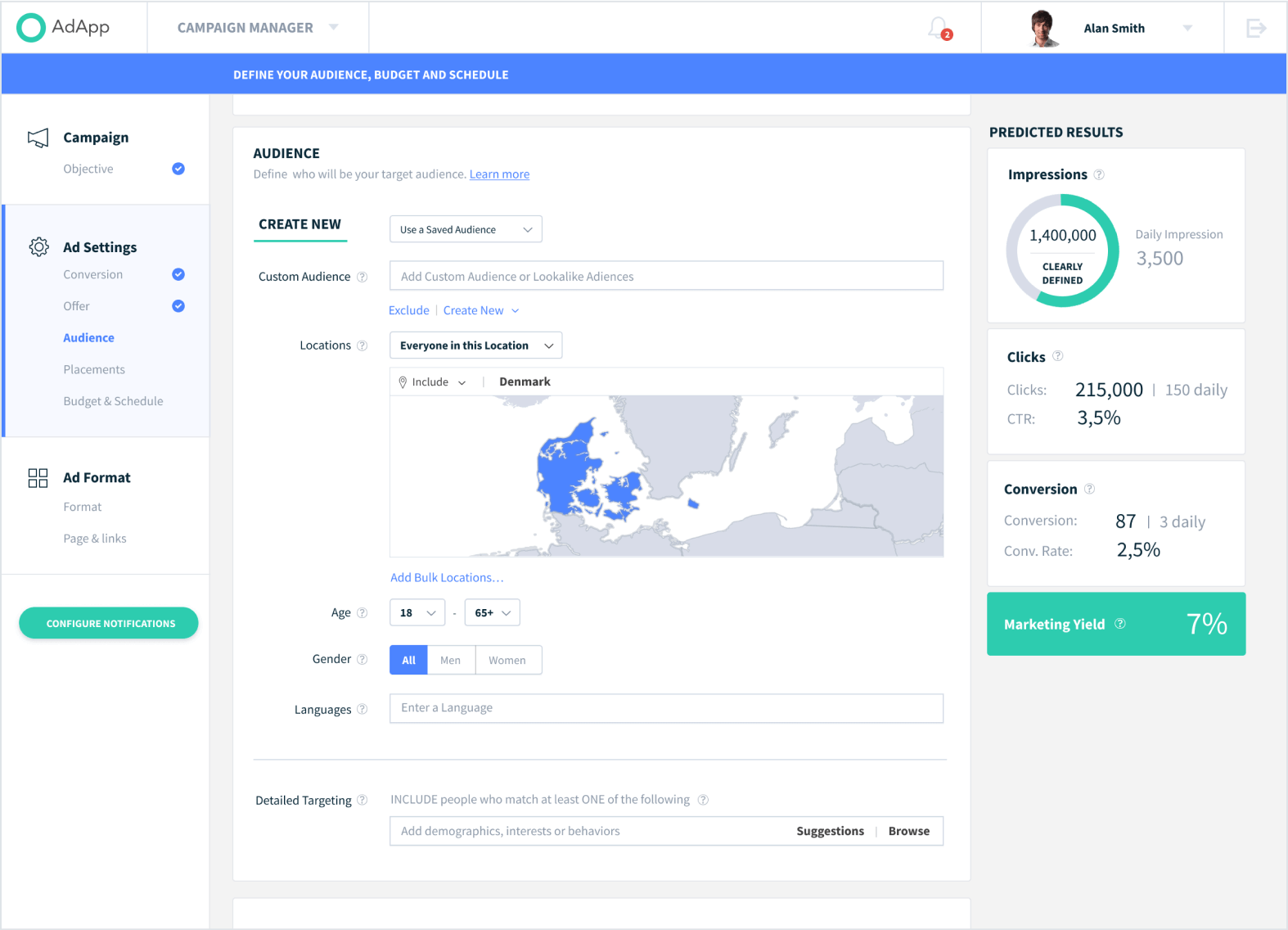
Use Cases:
- Customers: Embedded analytics is commonly used by SaaS companies that integrate other vendors’ analytics into their own product and provide it as a whole to their customers.
- Business Partners: It is also a popular solution among midsize companies and enterprises that use this type of analytics to drive business, assess operations, or mitigate risks by bringing data insights directly to their business partners (e.g., resellers, institutions, distributors, etc.).
- Departments: Lastly, embedded analytics has become increasingly popular for managing data and analytics for large-scale internal teams.
What Is Self-service Analytics?
Self-service analytics caters to organizations that want to promote data and analytics across their business. These organizations may be referred to as data-driven organizations or known for promoting data culture.
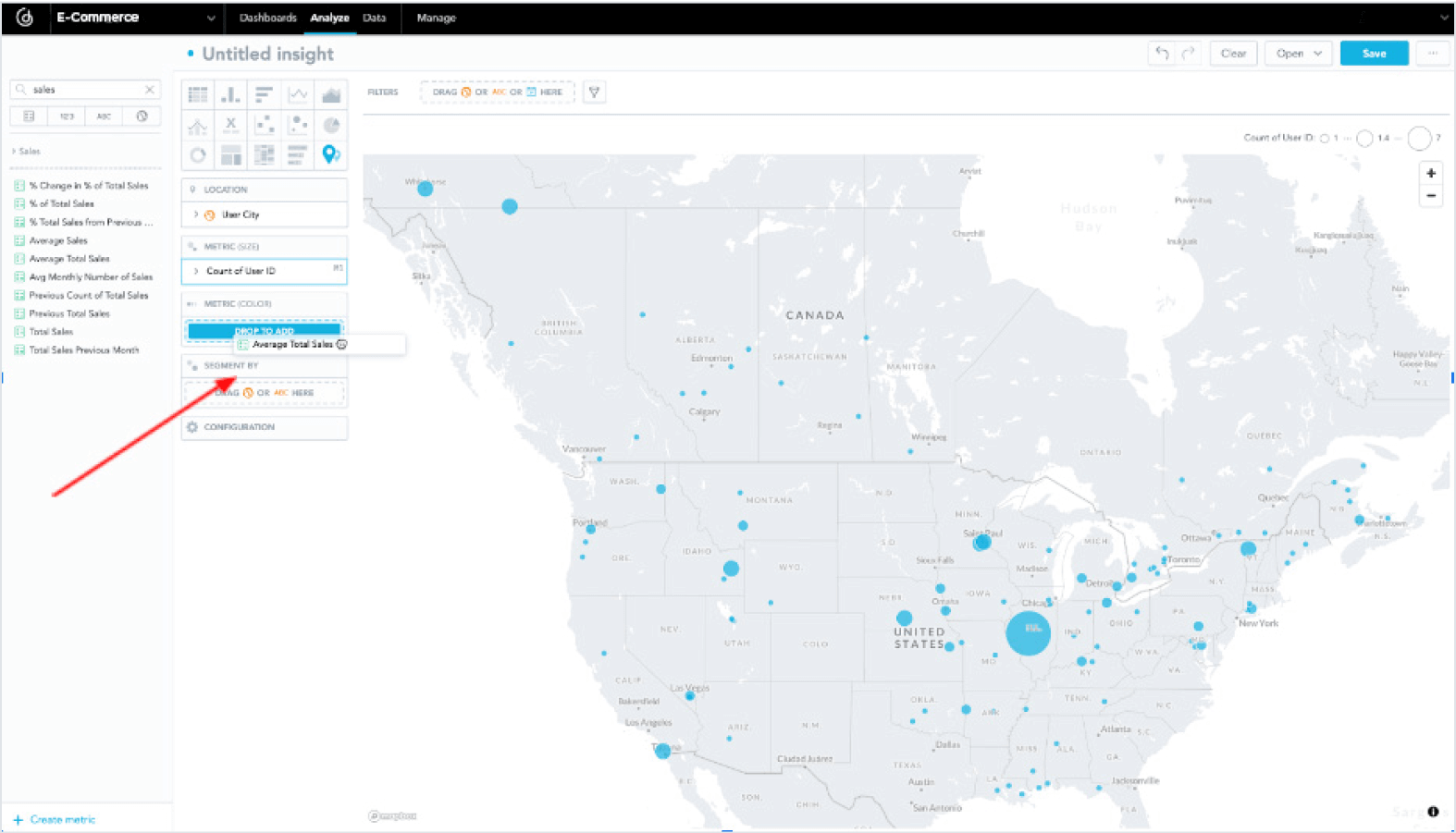
Before self-service analytics, the standard in the industry was that BI and data specialists would deliver data insights to business teams on demand.
With the increase of global data volume coinciding with the need to give business teams more access to data and reporting agility, self-service analytics has become more favorable.
Organizations now push to give their employees or business partners an analytics solution that allows these end users to perform ad-hoc analysis and data exploration on their own, while data teams and engineers focus on managing more complex cases or the whole solution.
Self-service analytics or self-service visualization serves this very purpose.
Use Cases of Business Users vs. Data Scientists
Business Users
With self-service visualization, business end users — users without a technical background or in-depth knowledge of data analytics — can create, customize, or drill into reports, charts, and dashboards. They are no longer dependent on data analysts, and they are equipped with data information to optimize their work and make informed decisions.
Data Scientists
In a broader sense, self-service analytics can also apply to data scientists and more technical users. The analytics platform could offer these users the interface and tooling to enable them to easily accommodate data models and metrics and work with the platform as needed.
Self-service Analytics Requires More Features for Success
It’s important to note that if the data analytics solution is intended to promote data culture with self-service capabilities across the organization, it needs to offer features for data operations teams. These features can include data governance, customization support, prototyping support, strong role-based access control, and a semantic layer for metrics management.
Part 2: How to Evaluate BI and Analytics Solutions
Beyond understanding critical terms such as BI, data analytics, self-service analytics, and embedded analytics, there’s a lot to consider when browsing options for BI solutions and eventually making a purchase decision.
The following is an abbreviated rundown of what to take into account if you plan on purchasing a BI and analytics solution from an external vendor.
Consider Use Cases and Corresponding Features
There are two major groups of use cases, which can then be broken down into more detailed use cases. We will only outline the differences between the two major ones as they are instrumental to understanding the set of features you will need.
Internal Analytics
The end users of internal analytics comprise teams and stakeholders within your organization. The end goal of this use case is to help those end users make more data-driven decisions — all based on facts, insights, and data analysis instead of guesswork.
External Analytics
Also known as distributed analytics or customer-facing external analytics, external analytics generally serves clients and partners. The underlying objective of this use case is to build analytics and reporting for these select individuals outside your organization.
Depending on whether your analytics use case is for internal or external deployment, you’ll need to examine a specific set of features more closely.
Recommended Features Based on Analytics Use Case
Self-service analytics:
Users without technical expertise can create their own charts and dashboards without assistance from data analytics specialists.
Internal Analytics
Must-have
External Analytics
Must-have
Interface white labeling and theming customizations:
Companies can change the analytics platform’s branding (e.g., colors, fonts, logos, etc.) to create a unified feel with their own brand and existing products.
Internal Analytics
Nice to have
External Analytics
Must-have (particularly for embedded analytics)
Full Interface embedding and advanced interface customization:
End users access analytics at the point of work and do not have to toggle between applications to get the insights they need.
Internal Analytics
Nice to have
External Analytics
Must-have for most cases
Architecture flexibility (scalability):
As companies grow, their analytics also need to accommodate a rising number of users, escalating costs, and overall greater user and data management.
Internal Analytics
Nice to have (however, for B2C companies experiencing growth, this is a must-have)
External Analytics
Must-have (limiting your own customers or partners could directly impact your business results)
Advanced security:
Security requirements affect the business of almost every globally operating company, including the security of data
Internal Analytics
Must-have (particularly if you are a global company or process sensitive data)
External Analytics
Must-have
Infrastructure and Data Source Connections
The analytics provider should offer a set of tools that connect to your data sources and ensure high performance.
Internal Analytics
Must-have
External Analytics
Must-have
Flexibility of deployment options:
With the increasing need to secure and protect data, it’s important to review and understand compliance stipulations regarding where your data will be stored at all times.
Internal Analytics
Nice to have
External Analytics
Must-have for most cases
Determine Your Evaluators
When evaluating BI and analytics solutions, there are three groups of stakeholders: business users, data engineers/analysts, and developers.
Below are the primary evaluation points of each group.
- Business users: Business impact/goals, easy-to-use interface, and drag-and-drop functionality
- Data engineers/analysts: Access to a strong underlying query language, composability and reusability of new metrics (metrics management), access to open APIs, and flexibility in terms of data connectivity
- Developers: Dedicated SDK with a set of low-code/no-code tools
Undergo the Evaluation Process
The evaluation process can be distilled into three major steps, culminating in your purchase decision:
- 1. Research and Discovery: Perform due diligence on analytics platforms — from assessing online comparison charts to heeding reviews from peers and analyst firms — in order to form a shortlist of top vendors. Remember your intended use cases and the accompanying recommended feature sets.
- 2. Product Demos: For each platform listed on your shortlist, request a demo call that offers a guided product tour and the opportunity to get specific questions answered (regarding features, integrations, and capabilities). A vendor’s sales and/or engineering teams will generally lead these demos, and the procedure may require several demos to satisfy an in-depth, technical evaluation.
- 3. Proof of Concept: A proof of concept is a customized demo prototype tailored to your primary use case and a sample of your data. This stage typically lasts a couple of weeks and is an opportune time to clarify any remaining questions as well as gain insight into future roadmaps and anticipated capabilities of the platform.
Part 3: Top Tools in the Market
The BI and analytics market is home to an abundance of different solutions, all seemingly similar to the rest.
In order to understand whether your BI implementation and wider data analytics strategy will be both a business and technical success, it is necessary to take a look under the hood to discover the major differences between each BI and analytics tool.
We have researched and compared major BI and data analytics providers on:
- Crucial features (architecture, dashboards, embedding, scaling and change management, data integration, data security and compliance, deployment options, and pricing. To see the results, download Comparing the Best BI Tools.
- Types of embedding (iFrame, SDK embedding, programmatic embedding, and plugins) deployment options, and pricing). To see the results, download Comparing the Best Embedding Tools.
If you have any questions about features or would like a free GoodData platform walkthrough, we are available to help you in Europe and the U.S. Simply request a demo to get started. Thank you.
Continue Reading This Article
Enjoy this article as well as all of our content.
Does GoodData look like the better fit?
Get a demo now and see for yourself. It’s commitment-free.