What Is Real-Time Analytics?
Written by Vanessa Wegner |
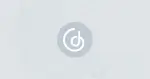
Table of Contents
Receiving your data and analytics in real time is an appealing prospect — from instant trend identification, to decisions that can be made without doubting the timeliness of your information. However, it’s not always apparent where real-time analytics provides the most value.
In this blog post, we’ll cover the definition of real-time analytics; what your data stack will need to achieve real-time analytics; and a few examples of who benefits the most from real-time data.
What Is Real-Time Analytics?
Real-time analytics dictates that data is processed and measured as it is ingested. This means that the components of your data architecture must also operate in real time — including data processing, data streaming, and any computation, logic, or mathematics that are part of your defined analysis.
Real-Time Analytics vs. Standard Analytics
Real-time analytics displays your acquired data immediately (i.e., as soon as the analytics are produced). Standard analytics are provided at regular intervals — hourly, daily, weekly, etc.
Real-time doesn’t always mean immediately: For some businesses, it can mean whenever the data is needed. Some use cases require updates every second, while some function perfectly with updates every 15 minutes.
Also, while data visualization principles for standard analytics also apply to real-time analytics, the latter is often delivered via alerts or notifications.
While these differences may seem obvious, the biggest ones are under the hood: in your data architecture.
On-Demand Real-Time Analytics vs. Continuous Real-Time Analytics
Real-time analytics often falls into two categories: on-demand and continuous. On-demand real-time analytics provides updated insights to users upon request. Meanwhile continuous real-time analytics updates your analytics automatically as new data is ingested.
Continuous real-time analytics can be especially helpful in process automation — such as fraud detection alerts, website management, and inventory management — but it will place higher demand on your infrastructure.
Want to see what GoodData can do for you?
Get a guided tour and ask us about GoodData’s features, implementation, and pricing.
Request a demoProcessing Data for Analytics: Stream, Micro Batch, or Batch Processing
Stream processing is when you process data as it is ingested by your system in real time, typically via a data streaming platform such as Apache Kafka.
However, real-time analytics isn’t for every business or use case. Near real-time analytics depends on micro batch processing, and it serves as a great alternative for use cases that can afford a few minutes’ delay.
Batch processing waits for data to accumulate before sending it through a data integration service such as Fivetran or Kafka. Data volume typically must hit a certain threshold or time period before the system will process it. This can take anywhere from an hour to several days depending on how it’s set up.
There are times when you need real-time analytics (e.g., transaction data or necessary, time-sensitive decisions), but most businesses will be better off with frequent data refreshes that don’t have to happen as the data is acquired.
How to Adopt Real-Time Analytics
The main challenge in adopting real-time analytics is figuring out your architecture. Real-time analytics and standard analytics have different requirements. To achieve real-time analytics, your entire data stack must be able to collect, process, and analyze in real time, at scale.
Adopting real-time analytics can be difficult for businesses that already have analytics infrastructure that is not suitable for real-time functionality — such as on-premises data centers, slow batch processors, or manual analysis tools.
As many businesses have shifted to the cloud for better flexibility, the modern data stack is still behind the needs of real-time analytics.
Requirements for Real-Time Analytics
- Big data capabilities: Real-time analytics systems need to be able to process massive amounts of data that often ebb and flow inconsistently.
- Change data capture (CDC): Many data warehouses cannot easily be updated, making it difficult to sync new data in real time. CDC can provide real-time data movement as new database events occur.
- Data streaming: Data streaming allows your data to flow continuously from its source, through processing, and into your analytics platform.
- Real-time data integration: Real-time data integration enables your architecture to collect and correlate data from different sources.
- Minimal to no data latency: Data latency is the time it takes to travel from your data warehouse to your analytics platform. For real-time analytics, the time between data ingestion and consumption needs to be near-instantaneous.
To reduce data latency and improve query speed, colocated data and analytics are a necessity. This means that the data and the function in which it is processed are on the same platform and in the same data center. Minimizing your data transfer by enabling direct queries to your data source can help improve response time.
Real-Time Analytics Use Cases
Real-time analytics isn’t always necessary, but for some organizations, it is the foundation for the value they provide. If you’re contemplating real-time analytics, consider the following questions:
- Should our decision-making rely on precise, in-the-moment data?
- Can we automate specific, immediate actions based on real-time information?
- Do we have a specific, valuable purpose for live status updates on our business?
If you answered yes to all of the above, real-time analytics has a valuable place in your data stack.
Some examples of specific real-time analytics use cases include:
- Flagging transaction fraud at the point of sale: With real-time analytics, payment companies can detect and automatically decline potentially fraudulent payments at the point of sale. This protects retailers and consumers, as well as the payment provider’s brand reputation.
- Enabling multiplayer online games: In games where players can compete or interact with each other online, real-time analytics are essential to the quality and accuracy of gameplay.
- Advertisement targeting based on in-the-moment consumer behavior: Consumer decision-making is fast — so getting your brand in front of them while they’re searching for a product or service is critical.
Real-time analytics is less important for transactions and decision-making that take place over a weekly, monthly, or longer time period. The same thing applies for tools that monitor aggregated data. For example, optimizing B2B sales processes, identifying real estate trends, and testing UX/UI decisions all will be better informed with data collected over a longer period of time.
How to Provide Real-Time Analytics
Beyond automation, real-time analytics is most helpful at the point of work — for instance, as embedded analytics or in a comprehensive dashboard, extended by a notification and alerting system for threshold changes. Real-time flags for trends, bottlenecks, or system failures can alert users to take action immediately, thus resulting in higher profits or lower risk for your business. Strong data visualization capabilities also come in handy for trends or changes that don’t trigger an automated response.
Summary
To give a brief summary, real-time analytics:
- Enables your business to ingest, process, and consume data as it is generated
- Allows both users and automation to make decisions and take action with in-the-moment information
- Requires a data stack that can process new data in real time
- Isn’t necessary for every use case, but can provide a significant competitive advantage in fast-moving industries
- Real-time analytics can be built together with standard analytics on a single platform to offer users a comprehensive data solution
Next Steps
GoodData offers automated, real-time query generation by way of its headless BI engine. Learn more by requesting a demo with one of our experts.
Want to see what GoodData can do for you?
Get a guided tour and ask us about GoodData’s features, implementation, and pricing.
Request a demoWritten by Vanessa Wegner |