What are Semantic Layers and Why Should Product Managers Care?
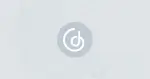
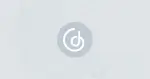
If you’re a product owner, you’re likely looking to integrate embedded analytics into your data product in a way that makes it usable by everyone in an organization. For those who are (which should be most product owners), you should be thinking about semantic layers. In my role as Senior Technical Product Marketing Manager, they’re something I’ve been thinking about a lot lately, and I’m not alone.
For those unfamiliar with semantic layers, think of them as a shield—or a layer, which is where the name comes from—between the user and the sheer volume of data that’s been collected. For a sales department, dozens of data points may be collected, most of which would make no sense to the user when seen individually. With semantic layers, those dozens of concrete data points are synthesized into a familiar concept—like “Q1 sales performance”—that is easier for the user to understand.
That’s just one reason why you should be thinking about semantic layers. As the use of embedded analytics becomes more widespread, they make it so non-technical users don’t have to understand the complexity of the schemas, tables, and columns in data sources. As a result, users can conduct ad-hoc data explorations in a way that makes sense to them, without having to dive into the underlying data structure or programming or data language.
Semantic layers also remove bottlenecks by enabling users to answer questions themselves without relying on other teams to respond to queries. While this is great for the user, it has the added benefit of freeing up your IT resources, so you don’t spend a bunch of time answering users’ queries, which may vary in complexity and difficulty in answering.
By ensuring that the data is properly handled and that insights come from a single point of truth, users can always be sure they’re getting the correct answer from a reliable source, which leads to better data governance. And in the event that the original author of the semantic layer leaves the organization, the semantic layer can hold onto their knowledge, storing details about where the data lives and how to query it correctly so things can continue to run smoothly. From a security perspective, organizations may need to know who accessed which data and when or may need to allow only authorized users to see certain data. Semantic layers can track all of that information and confirm authorization, so organizations can be confident that they’re in compliance with any requirements, legal or otherwise.
Of course, semantic layers are continuing to evolve, thanks to AI and machine learning and other requirements for large-scale embedded analytics data products. I’ll be diving into how those capabilities will affect the future of semantic layers in my next blog post.
As analytics becomes more pervasive, your user base will expand—or may have already expanded—dramatically. Increasingly, when building analytical applications, you’ll need to think about how you’ll translate technical representations of data into business needs. For those considering buying a solution, realize that not all semantic layers are created equal—I’ll talk more about that in an upcoming blog post as well. After all, none of us can afford to lag behind competitors whose applications make it easier to use data effectively.
Want to see what GoodData can do for you?
Get a guided tour and ask us about GoodData’s features, implementation, and pricing.
Request a demo