Measuring the (Business) Success of AI
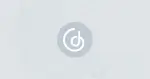
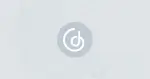
When they think of Artificial Intelligence (AI), most people picture a future world that Hollywood and technology visionaries have painted for us, filled with androids, autonomous vehicles, and other futuristic toys. Here in 2017, things are moving just as quickly in the boardroom where business leaders are scrambling to integrate AI into their enterprises. Forrester Research recently predicted that investments in AI will increase by more than 300 percent in 2017 compared with 2016. Additionally, IDC estimates that the AI market will grow from $8B in 2016 to more than $47B by 2020.
It’s getting to the point where AI is looked at as a “must have” for businesses of the future. I worry, however, that organizations that rush to shoehorn an AI element into their models for the sake of relevancy without a solid strategic plan are doomed to fail. In fact, a study of businesses that are already using these systems found that only 1 in 10 believe they’re getting the results they’d hoped for. There is still a huge gap between research into AI and delivering actual tangible business results in the real world, with a recent Forrester survey showing that while 58 percent of companies are researching AI, only 12 percent were actually using AI systems in their businesses.
As enterprises from every industry pour money into AI, it’s important to remember that this is an early adopter market, and the business impacts of many of these implementations are still very much in the “wait and see” phase. But even as organizations rush to implement AI strategies to align with their own digital transformation journeys, it’s important that we shift the conversation around AI from simply “everyone needs it” and start focusing on how to truly drive value from and measure the success of these initiatives.
Why not try our 30-day free trial?
Fully managed, API-first analytics platform. Get instant access — no installation or credit card required.
Get startedMeasuring the business success of AI is a complex question. For some companies, these solutions are a labor and cost savings measure and should be analyzed accordingly. Other implementations are tied to revenue generation, while some might not fit so nicely into “hard” metrics, so how can companies really know if things are working? Or, if they’re just starting out, how can they at least tell if they’re heading in the right direction? I would argue that any evaluation of AI deployments should include three core areas:
Does your solution increase productivity through the automation of routine decisions and augmentation of decision-making workflows?
Automating routine decisions that fall under fairly rigid, established parameters is a perfect use case for AI. Imagine how powerful it would be if, rather than having to self-perform data analysis, workers were given access to tools that automatically present them with simple, reliable and machine-accurate recommendations for the next business actions they should take.
Radical advances in computing power, predictive analytics, machine learning and artificial intelligence have opened the door to a new generation of analytic tools that can instantaneously pull actionable insights from complex data sets and automatically surface them as recommended business actions, where and when they are needed most. This saves time, and frees up your more expensive talent to focus on strategic tasks.
Measuring success in this regard means looking for increased productivity and improved decision making. Loan officers, for example, will soon rely on automated insights to make the most risk-averse decisions possible. While algorithms are already being used to recommend approval or denial of loans, these are antiquated systems. Advances in machine learning mean these systems can be augmented and enriched to include unstructured data from social media, IoT, buying behavior and more to paint a much more accurate picture of applicants far more quickly, dramatically improving performance.
Will incorporating AI into your business model lead to a reduction of errors & biases?
Humans, no matter how intelligent, are prone to error and bias when it comes to data analysis. Fortunately, AI is ideal for cutting down on servicing mistakes, human-driven interpretation errors, and incorrect financial projections.
Machines are getting better at extracting insights from complex data than humans are. JPMorgan, for example, implemented a machine learning solution called COIN that completes in seconds work that used to take a team of lawyers thousands of hours to complete. By automating the task of interpreting commercial-loan agreements, COIN reduces errors in loan-servicing while sparing JP Morgan’s legal team more than 360,000 hours of work each year, saving the company millions of dollars.
Can you leverage AI to deepen your customer relationships with new client-facing products?
Again, JPMorgan are leading the way here by offering some of their AI technology to their clients later this year. Doing so will allow these clients to access balances, research and trading tools and bypass salespeople and support staff for routine information, adding value to JPMorgan’s offerings and strengthening their client relationships.
This is an excellent example of how, if executed thoughtfully, incorporating AI into your business model can benefit your customers in ways that facilitate a better customer experience, better relationships, and create opportunities for new revenue streams. And if those three things are happening, your AI program is already a success.
This article originally appeared in Information Management.
Why not try our 30-day free trial?
Fully managed, API-first analytics platform. Get instant access — no installation or credit card required.
Get started